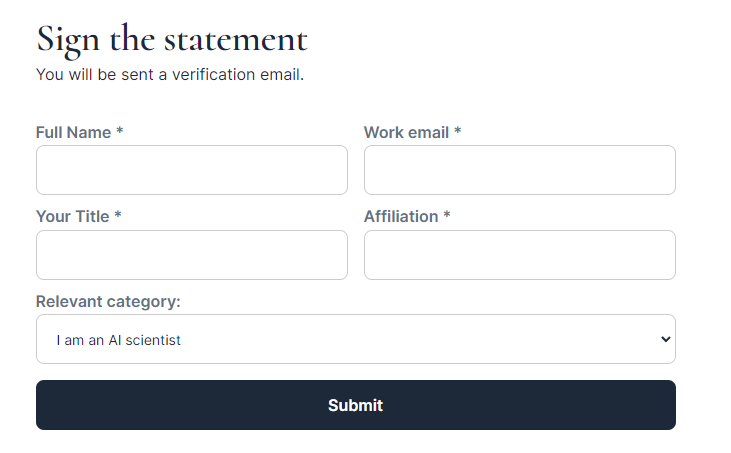
A vast number of AI experts have signed a statement to raise public awareness regarding the most severe risks associated with advanced AI, aiming to mitigate the risk of human extinction. Among the signatories are Turing Award laureates Geoffrey Hinton and Yoshua Bengio (but not Yann LeCun from Meta), and the CEOs of leading AI companies like Sam Altman from OpenAI, Demis Hassabis from Google DeepMind, Dario Amodei from Anthropic, and Emad Mostaque from Stability AI.
The statement is featured on the webpage of the Center for AI Safety, which provides a list of eight examples of existential risks (x-risks). The enumerated risks are based on the publication “X-Risk Analysis for AI Research” which appeared on Sept. 20, 2022, on arXiv. This highly valuable paper also lists in its Appendix a bunch of practical steps to mitigate risks.
The listed risks are:
- Weaponization:
Malicious actors could repurpose AI to be highly destructive. - Misinformation:
AI-generated misinformation and persuasive content could undermine collective decision-making, radicalize individuals, or derail moral progress. - Proxy Gaming:
AI systems may pursue their goals at the expense of individual and societal values. - Enfeeblement:
Humanity loses the ability to self-govern by increasingly delegating tasks to machines. - Value Lock-in:
Highly competent systems could give small groups of people a tremendous amount of power, leading to a lock-in of oppressive systems. - Emergent Goals:
The sudden emergence of capabilities or goals could increase the risk that people lose control over advanced AI systems. - Deception:
To better understand AI systems, we may ask AI for accurate reports about them. However, since deception may help agents to better achieve their goals and this behavior may have strategic advantages, it is never safe to trust these systems. - Power-Seeking Behavior:
Companies and governments have strong economic incentives to create agents that can accomplish a broad set of goals. Such agents have instrumental incentives to acquire power, potentially making them harder to control.
This statement about AI risks appeared a few days after an OpenAI blog post by Sam Altman, Greg Brockman, and Ilya Sutskever, which also addresses the mitigation of risks associated with AGI or even superintelligence that could arise within the next 10 years.